About Between Data and You
This site will provide you with a behind the scenes look at how analytics shapes a number of things in our daily lives.
Specifically, we will be examining how businesses use data to their advantage, and how we, as consumers, are affected.
The first section of the site defines WHAT analytics is and how it has evolved in response to rapidly developing technology. It breaks down the different types of analytics and provides you with a timeline that displays the appearance of relevant terms and technologies. The goal here is to develop a high-level understanding of terminology that I will use moving forward, although there is always the option to explore further. Additionally, I want to draw attention to points where thinking has shifted as a result of technological advancement. Follow how analysts' methods and roles have adapted!
The second section explains WHO an analyst is. It defines their different responsibilities and provides examples of tasks. It lists relevant skills and the analytic process. See how an analyst approaches every problem she is presented with! This section also includes examples of the types of questions that analysts are asked to answer.
The goal of the third and final section is to show WHY analytics matters to you. Here, we will explore how analysts have made decisions about the products and services you use every day. This section is rooted in examples and stories from companies about how analytics has changed the way they do business.
click the underline to see how I will be defining analytics
I will pull back the curtain and reveal the analytics that drive everything around us.
About Me
I am an incoming entry-level analyst whose interests are focused around problem solving and critical thinking.
I am less concerned with the technical logistics of analytics and drawn more towards the methodologies and thought processes behind data-driven decisions.
I want to learn how successful analysts think through challenges. The first step in achieving this goal is to develop the ability to break down problems and questions and recognize how to best approach them. To do this, I need to understand the specifics of this broad, general field. What is analytics? Next, I need to identify what my role is. Who are analysts? Finally, I need to pull from the knowledge of what others have successfully done before.
Some of the goals of this site are personal, as I am attempting to expand my knowledge and consequently better my analytical skills. But the remaining goals expand outward and are relevant to not only me, but also to everyone who visits this site. Analytics inform almost everything in our daily lives.
I hope to shed light on the ubiquity of analytics. l also want to draw attention to the ways in which analysts are involved in business processes that typically go unseen. There are people behind many of the decisions that we take for granted, and I want to underscore their importance.
About You
You do not need to have a background in economics, computer science, or math to explore this site. Here, I have simply aggregated the information that I found to be useful in my own understanding of analytics. I have also included the most relatable references and examples.
By exploring businesses' focus on data-driven decisions, we will gain the terminology and knowledge necessary to join larger conversations about business methods and data. Moreover, we will become informed consumers, able to see how our actions contribute to a larger analytical network.

WHAT IS ANALYTICS?
ANALYTICS
Logical analysis. Computational analysis. Data analysis.
ANALYSIS
An examination. Separating something into its elements. Studying the nature of something. Determining essential features and their relations.
Analytics is the analysis of patterns or irregularities in data points to solve problems, answer questions, spot errors, increase efficiencies, and make decisions. Its modern form is a response to an increase in “big data,” which is comprised of all of the data that is collected about and around us.
Think of your Fitbits and social apps. Think of online advertising and online paths. Think of all the forms you’ve ever filled out in waiting rooms that have moved from physical files to databases. Think about GPS location and customer service robots and employee satisfaction surveys.
Everything with a digital footprint is tracked. And all of this data is being kept, thanks to technologies that have increased storage capacity.

There are three main types of analytics that leverage this accumulated data differently:
Hover to see definitions. Click the questions to see examples.
Descriptive Analytics
Analysts aggregate businesses' data to determine the best next steps. They focus on figuring out what has worked, what has failed, and why.
Predictive Analytics
Analysts use past trends to predict what will happen in the future. They create models and simulations, which allow companies to anticipate losses and set realistic goals.
Prescriptive Analytics
Analysts use data to identify and solve business problems. They create and then relay data-driven recommendations for optimization to management.
Analysts have employed these types of analytics at different points in analytics' brief history. Currently, all types are a part of an analyst's role.
The focus has shifted away from descriptive analytics, and now businesses are working to further develop predictive and prescriptive analytic capabilities.
HISTORY &
EVOLUTION
The 2000s marked the start of a sprint to gain a competitive advantage on analytics. This was the era of “big data,” which was characterized by online, externally sourced data that was often unstructured and extremely large. This data was processed with more advanced technologies that allowed the analysis to happen much quicker than it had before, but the main challenge was still data management. More complicated questions could be asked, and analysts focused on predictive analyses.
There was an emphasis on complex and usually interactive visuals or models that allowed stake holders to filter and tweak the reports to answer their specific questions. Analysts and executives worked more closely, as analysts became involved in every aspect of companies in order to shape the business based on data.
Using analytics to make better business decisions appeared in the 1950s, but it didn't become a norm until the 1990s. Still, only large companies could afford to make the investments necessary to develop this aspect of their business.
Analysts aggregated and reported internal data that described production processes, sales, and customer interactions. Most of their time was spent preparing the data, and the analysis was tedious and slow.
This era produced a more objective understanding of business operations and marked a switch from mainly experience or intuition-based decisions to fact-based ones. Descriptive analytics was employed to understand how things were preforming, but there was still a disconnect between analysts and executives, so analysts’ recommendations were not always taken seriously.
(hover over terms to learn more, click underlines to view in-depth external articles)
1958
"business intelligence"
IBM researcher uses term to describe the use of facts to reach business goals
1960s
decision support systems
a computer based informational tool that aid in organizational processes
1970s
"data warehouse"
SQL
Structured Query Language: a programming language used to manage and extract data from a database
1977
1989
1996
"business intelligence," again
umbrella term to describe methods to making business decisions by using fact-based support systems
1998
enterprise resource planning
describes the management of business processes integrated with technology and softwares
1999
"Internet of Things"
2002
"Chief Data Officer"
2003
"Moneyball"
Michael Lewis publishes book about Oakland A's use of analytics to manage and win
2000s
"machine learning" & "artificial intelligence"
"streaming analytics"
a greater emphasis on analyzing data in real time
Analytics 1.0
Analytics 2.0
Analytics 3.0
2004
SAS
Statistical Analysis System: version 9.0 that includes Text Mining and a user-friendly interface
LinkedIn data products
mobile analytics
more products that record and analyze mobile user behavior enter the market
2011
2018
natural language
analytics software shifts towards natural language for queries as opposed to programming
2006
"cloud computing"
describes shift from using and storing software and files on a desktop to the web
2010
International Institute for Analytics
2014
"Chief Analytical Officer"
We are in the very beginning stages of this era of analytics. It is characterized by a use of analytics to develop products and services. This notion is distinct from Analytics 1.0 or 2.0, in which analytics was used to improve internal business decisions. Now we’re taking it one step further and creating based on the data.
Analytic capabilities are being embedded into products and services to better understand consumer and market needs. There is a greater integration of analytics, products, and business. Machine learning is further developed and utilized, and the responsibilities that analysts had in 2.0 are automated. These aspects of 3.0 highlight prescriptive analytics as playing a vital role in competitive advantage.
This increased automation and integration reduces analysts' need to "fix" things. Their focus will shift to a different kind of question: what does the market want? Analysts will look to data in order to spot opportunities and answer. of
What's Next?
It is now necessary for companies to develop their analytic capabilities in order to be competitive.
So how is this method of thinking being practically employed? And who is behind the decisions being made?
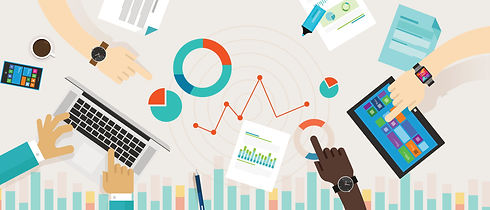
WHO ARE ANALYTS?
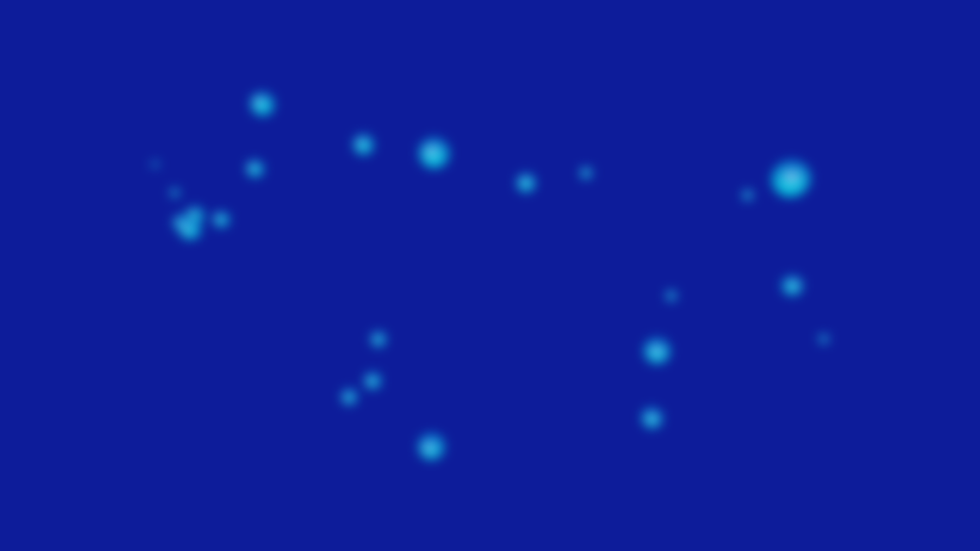
It is easy to focus on the technology that has enabled analytics to evolve to its current point.
In this section, I will pull apart some of the processes that we take as given and explain how analysts interact with data.
What are they tasked with?
Analysts' responsibilities can be broken down into three categories that coincide with the three types of analytics presented above:
Click the icons to see an example.
Reporting
Analysts take data that data scientists have extracted and structure and aggregate it to report performance.
There are many questions that management could present to an analyst. These questions could directly state the task ahead or it could be the case that the analyst has to figure out what she's being asked to do.
See if you can determine which tasks best fit the question. Hover to see the answer.
"What should we do with all of this user data from our app?"
"Are there any issues with losses?"
"How should we spend $1,000,000?"
"Why is this statistic so low?"
"When should we launch this?"
"How is the new feature performing this month?"
"What will next month look like?"
"How should the next version be different?"
"Why aren't customers following through?"
You'll notice that most questions involve projects with multiple elements. One question will usually produce 10 more that the analyst has to address.
Reporting allows analysts to dig into statistics at more granular levels until problematic sources are found. This type of work involves diving deeply to uncover meaning behind the numbers. So it might be a task to create a report, but projects generally involve the use of a regularly generated report to inform their recommendations.
Larger, more complex projects usually start with reports and end up cycling through different models and development stages until all associated questions are answered.
All of these projects have one thing in common: PRESENTATION.
At the end of all of these tasks, when an answer has been found, analysts have to translate their findings to management and different stakeholders. Using visuals and only the most relevant numbers, analysts have to support their claims in a clear concise manner. They have to recognize their audience and tailor the conversation accordingly.
Most stakeholders don't have a background in data, and it's the analyst's job to translate the numbers and make them meaningful.
So what process are these questions cycling through?
And how do they come up with answers?
PROCESS OF ANALYSIS

There are steps that every analyst follows when approached with a project.
These steps are usually applicable to several elements of the project, so the analyst cycles through this process for different areas of interest (the sub-questions that the initial question invites). Frequently, an analyst completes a step that will force them to backtrack to a previous point and redo the work.
The process is run through until all sub-questions and elements are addressed and analyzed. Then, the analyst can make a strong recommendation backed by the data.
1. Decide on Objectives
Once presented with a project, the analyst needs to determine what the end product looks like. What are the goals?
2. Identify Relevant Metrics
A metric is a unit of measurement. Data is aggregated into different metrics such as people per household or average wait time. The analyst must know which metrics she should pay attention to.
3. Explore the Data:
What is Happening?
If the project is a direct question or task:
The analyst needs to get a handle on the data. What do the metrics look like now? How have they looked in the past, and can I make a guess at how they'll look in the future? Is there a metric that I could create that would better show what is happening?
If the project presents a problem:
The analyst wants to identify the root cause. She needs to go through different reports to find irregularities and telling trends. Once she can narrow her focus to one problematic business unit or metric, she is better equipped to move towards a solution.
4. Identify Possible Recommendations:
What Should be Happening?
Once the analyst knows what is happening or what will happen, she needs to brainstorm what can be done about it. What are ways to move forward or adjust that will benefit the company?
5. Narrow Down to the
Best Recommendation
To do this, the analyst must consider the business units that the recommendation will effect. She will look at plausibility through the lens of profitability in order to pick one recommendation.
Additionally, she needs to identify which business units she will be presenting to. Which managers asked her to complete this task? Who are the stakeholders? She then needs to tailor her recommendation so that all parties involved understand how she arrived at her conclusion.
She needs to translate her findings so that others can clearly see how her recommendation ties to their interests.
The length of the process and the number of times different steps are backtracked and gone over again are completely dependent on the project.
An analyst could be doing something small and simple, like creating a report for the roll-out of a new product in order to assess its performance. But she could also be addressing why sales are down, a complex question with a lot of moving parts and implications.
So now knowing what analysts do and how they do it, we want to explore why they do it and why it matters to us, the consumers.
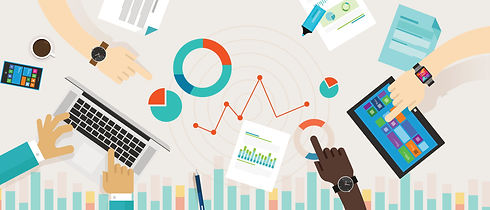
WHY SHOULD WE CARE?
Every company in every industry employs analysts. Their projects and recommendations are integral to the companies' operation.
In making those operations run smoothly, analysts are doing us, the consumers, a service. They fix profitability problems and create products that allow us to consume things at the prices we do. They put more value into the things that we buy. But we usually don't know it.
In the slideshows below: see how we consume, and click through to learn how analysts play a role in that consumption.
You have now seen behind the curtain. You understand how businesses use data to drive their decisions and ultimately increase their profits. You know that analysts exist, and you know how they work.
Now that you know, what should you do?
You have two options:
You can continue to consume as you did before. You can allow the analysts to do their jobs behind the scenes. You can decide to not be an active part of analytics, although you will always be a passive one. Companies will continue to increase their efficiency. Analysts will continue to be needed to solve and anticipate problems. And technology will continue to allow for more data to be collected and analyzed in more sophisticated ways.
OR
You can choose to create more purposeful data. You can start looking at the decisions you make as bits of data that companies are going to use to their advantage. You can consume more consciously, in order to create data that displays real interests and behavior that you can be proud of. You can use this knowledge to your advantage.
Sometimes, we think that our online habits and actions are discrete and distinct from one another. We can't imagine that there is any link between the things we search for on a Friday and the content we post on a Monday.
But now you have seen the link. You have learned that analytics is the field that takes these aggregated habits, actions, data points, and turns them into actionable recommendations. These recommendations lead to increased efficiency and product improvement/creation, all for the purpose of driving profits.
This ecosystem of data happens all around us, all of the time. Whether we want it to or not. And we can either: 1. ignore it, or 2. acknowledge it and take some control.
The part that we play in this ecosystem is as the consumer. As a consumer, the actions that we can take can be summed up into data creation. Of course, what you purchase is a data point. But you also must recognize that what you browse for, what you like, what you post, what videos you watch, which platforms you use and how you use them are also data points.
These are the bits of information that we create, and we can either choose to do it absentmindedly or purposefully. I encourage you do so with purpose.
If you choose to create more purposeful data, as I did, you will spend less time online shopping. You will only look up the things that you need, and you will browse less. You will recognize that the advertisements you receive in your inbox and on your Internet browser are the direct result of your own search history. You will slowly make it so that these ads are truly personal and relevant.
You will optimize your social media. You will delete follows and followers that aren’t directly in line with the online persona you want to display. You will “like” only the things you truly like, and you will be a part of only the groups and communities where you feel as though you actually belong. Your online profiles will accurately reflect the current you.
You will download fewer apps. You will sign up for fewer newsletters and email lists. You will use more loyalty programs. You will gain more rewards. You will utilize your products more efficiently, and you will make better use of data products and data driven products.
Once you understand some of the ways in which your data is being used as a consumer, you’re able to take the process of analytics into your own hands. You know what analysts do, how they do it, and why. Everything starts with that initial set of data. You have the ability to change those outcomes based on the data that you create. So if you create your data mindfully and with purpose, you can increase the efficiency of the whole system. As the consumer, you will ultimately reap those rewards.
NOW WHAT DO YOU SEE?
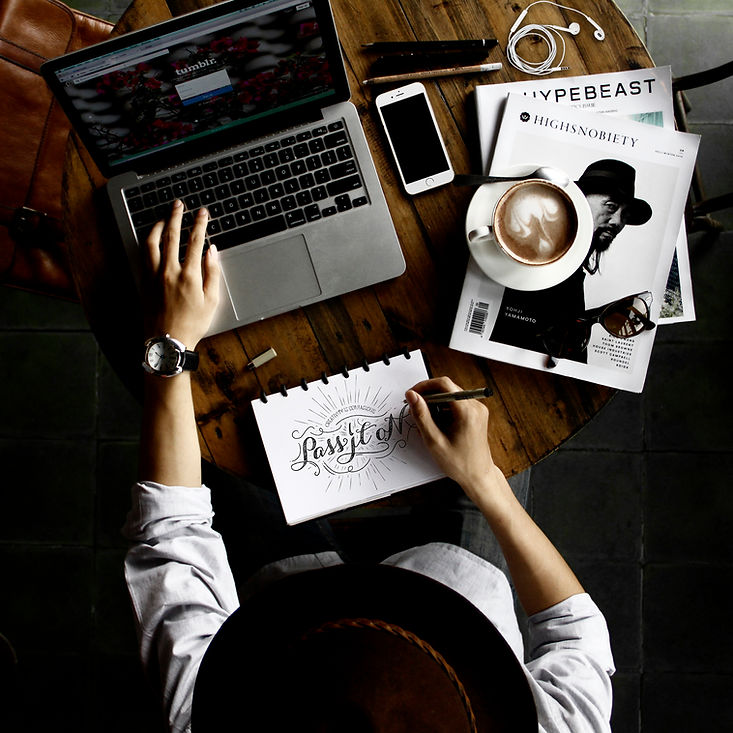